Introducing the AI Stages of Adoption: A framework for understanding AI readiness in your business
Earlier this week, I got the opportunity to speak at our Amazon Web Services (AWS) office in London, to an audience of Private Equity firms and their portfolio company executives about driving value creation through AI and data. The presentation focused on how Private Equity firms and their portfolio companies can drive value creation through AI and data. I also took the opportunity to introduce a new concept called the AI Stages of Adoption (AISA), which is designed to help organisations assess their maturity and readiness for AI adoption.
The AI Stages of Adoption (AISA)
Around 2015 AWS developed the concept of the Cloud Stages of Adoption (SoFA) which became an easy way to understand where organisations were in the journey to the cloud. I’d been thinking about a similar mechanism for AI adoption since the announcement of ChatGPT, so last year as I began scaling generative AI enablement to AWS leaders ahead of re:Invent, and I decided to finalise the idea of the AI Stages of Adoption (AISA) and make it available.
In this video, you’ll see that AISA is represented on a graph showing investment (money, time, talent, etc.) along the X-axis and value (tangible and non-tangible) realised on the Y-axis. The line represents a linear journey of adoption. You’ll also notice some dotted lines with different levels of investment and value realisation. This is conveying that multiple AI and data initiatives are running at the same time, and can deliver vastly different value outcomes relative to their investment.
AISA outlines five stages of AI adoption:
- Experimenting: Organisations explore and test new technologies through pilot projects or small-scale implementations.
- Adopting: Successful experimentation leads to wider adoption, integrating technology into existing processes and establishing governance structures.
- Optimising: Organisations refine processes, customise the technology, and identify areas for improvement to maximise benefits.
- Transforming: Technology becomes deeply embedded, transforming operations and leading to the redesign of processes, roles, and business models.
- Scaling: Full embrace of the technology, focusing on scaling its adoption across the entire organisation or ecosystem to drive growth and competitive advantage.
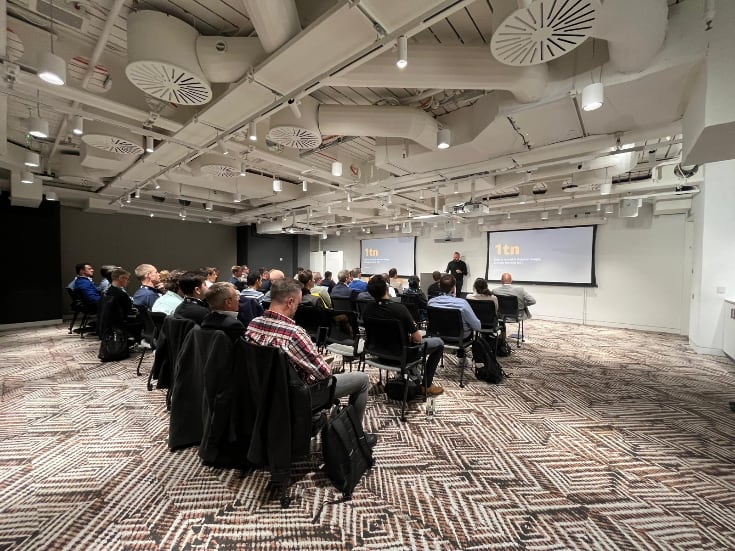
The Eight Disciplines of AI
During the presentation, I discussed the eight current disciplines of AI that offer multiple opportunities for transformation across different business use-cases:
Machine Learning: Training algorithms to learn from data and make predictions or decisions without explicit programming. Examples include predictive maintenance for industrial equipment and customer churn prediction in telecommunications.
Deep Learning: A subset of machine learning that uses artificial neural networks with multiple layers to learn from vast amounts of data. Applications include medical image analysis and autonomous vehicle perception and control.
Natural Language Processing (NLP): Enabling computers to understand, interpret, and generate human language. Common applications include sentiment analysis and automated summarisation of legal documents.
Computer Vision: Enabling computers to interpret and understand visual information. Prominent applications include facial recognition for security and defect detection in manufacturing.
Reinforcement Learning: An agent learns to make decisions by interacting with an environment and receiving rewards or penalties for its actions. Uses include dynamic pricing optimisation for e-commerce and robotics.
Robotic Process Automation (RPA): Using software robots to automate repetitive and rule-based tasks, freeing up human workers for more value-added activities. Common applications include automated data entry and intelligent form processing.
Cognitive Computing: Simulating human thought processes and reasoning for more natural and intuitive interactions between humans and machines. Applications include personalised learning experiences in education and intelligent virtual assistants.
Generative AI: Creating new content such as images, text, or music based on learned patterns and rules. Applications include creating synthetic data to train machine learning models and generating multiple design variations in product design.
I highlighted the importance of data as an enabler of value creation, discussing the proliferation of data in business, data silos, shadow operations, and comparing synthetic and real-world data. Proposing that with that data, organisations should be looking at all forms of AI, not just the current poster child of AI; generative AI.
AI and data innovation can influence strategic business outcomes such as increasing revenue margin and profit, catalysing innovation, enhancing customer experiences, improving operational efficiency, reducing organisational risk, and increasing sustainability. I shared statistics to demonstrate the value of data and insights-driven businesses, including:
- The growth rate of insights-driven businesses is 30% (Forrester).
- Data and insights-driven businesses are 23x more likely to acquire customers, 6x more likely to retain customers, and 19x as likely to be profitable (McKinsey).
- Data-driven businesses are 58% more likely to beat their revenue goals than non-data-driven companies (Gartner).
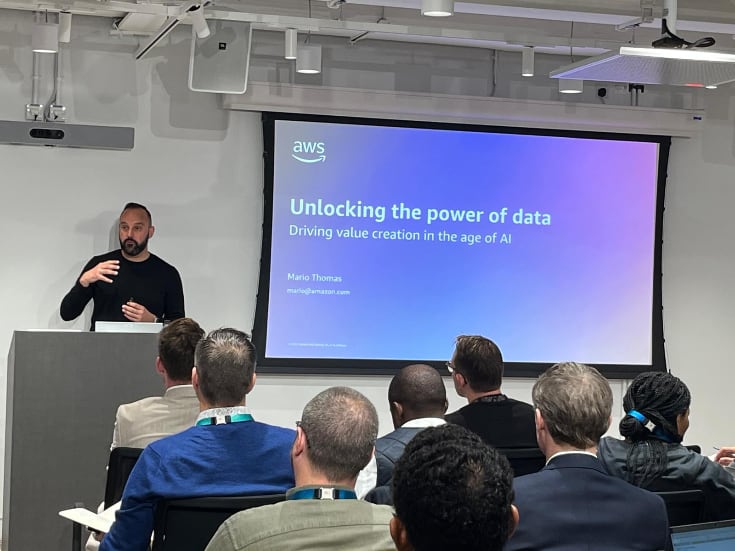
Preparing for AI Adoption
Similar to my LSE lecture last week, this section addressed the practicalities of AI implementation and data strategy, focusing on potential risks, legal considerations, and the crucial role of Board oversight. It emphasized the need for a robust governance framework to manage AI adoption responsibly. Key concepts included:
- The need for governance of AI: The importance of Board ownership of AI governance, ensuring that AI initiatives align with the organisation’s strategic goals and ethical standards.
- Steps to create an AI centre of excellence: Establishing a dedicated team to drive AI projects, foster collaboration, and ensure best practices.
- Areas of concern for Boards: Issues such as data privacy, security, bias in AI models, and compliance with regulations.
- Key questions for AI project leaders: Critical questions Boards should ask to ensure effective oversight and accountability in AI initiatives.
- Addressing hallucinations, hypnosis, poison tokens, prompt injection, and bias:
- Hallucinations: The phenomenon where AI generates incorrect or nonsensical information. Ensuring rigorous validation and verification processes can mitigate this risk.
- Hypnosis: The subtle influence AI might exert on decision-making processes. Maintaining human oversight is crucial to avoid unintended manipulation.
- Poison Tokens: Data points that can corrupt AI training data, leading to skewed or harmful outputs. Implementing robust data cleaning and monitoring practices is essential.
- Bias: The inherent biases that AI models can perpetuate. Regular audits and diverse training data can help in reducing bias and ensuring fair AI outcomes.
- Prompt Injection: A method of injecting harmful prompts into AI input to manipulate its responses, similar to SQL injection in databases. Ensuring secure and monitored inputs can prevent such attacks.
I also took questions from the audience on the topic of the establishment of an AI Centre of Excellence - something I am strongly of the view belongs alongside the Board and not in IT or within any cloud centre of excellence or cloud business office.
I wrapped up with some thoughts the low barrier to entry with AI, the misconception that you have to be ‘data perfect’ to get started, and how you can quickly get started with generative AI with Amazon Q Business with little friction, low fidelity in data used to train Amazon Q, and realising almost immediate business value.
And Finally
Finally, I shared a Amazon PartyRock app I wrote specifically for this presentation called the Money for Old Rope Machine (MORM). MORM allows users to propose an industry and a type of data, and will then offer a number of use-cases which could be addressed through AI (give MORM a try here)
About the Author
Mario Thomas is a transformational business leader with nearly three decades of experience driving operational excellence and revenue growth across global enterprises. As Head of Global Training and Press Spokesperson at Amazon Web Services (AWS), he leads worldwide enablement delivery and operations for one of technology's largest sales forces during a pivotal era of AI innovation. A Chartered Director and Fellow of the Institute of Directors, and an alumnus of the London School of Economics, Mario partners with Boards and C-suite leaders to deliver measurable business outcomes through strategic transformation. His frameworks and methodologies have generated over two-billion dollars in enterprise value through the effective adoption of AI, data, and cloud technologies.